Thanks to the ubiquity of smartphones and the availability of wearable technologies, large-scale data collection is on the rise. People log their steps with commercial products such as Fitbit and keep track of their activities and locations using apps like endomondo. Gordon Bell [1] went even further and made it his mission to attempt to record his entire life: images, sounds, videos as well as personal documents. He pioneered the trend towards lifelogging applications that has been supported by research projects such as Microsoft’s SenseCam [2]. Whereas technologies such as Fitbit are aimed at collecting quantitative data about movement and health to set incentives for improvement, the SenseCam serves as memory aid by automatically capturing a digital record of the wearer’s day.
Further, attempts have been made to recognize activities, such as walking, running or sleeping by making sense of smartphones’ accelerometer data [3]. The quantified life is not limited to physical activities only. Kunze et al. introduced a system that used eye tracking in combination with image retrieval techniques to track what and how much people read in their daily lives, thereby creating the wordometer[4]. Kunze describes this development of tracking such activities for the mind as the ’trend towards a cognitive quantified self’.
Taking together the logging of physical and mental activities and combining them with a life log of moments and memories we are getting closer to a holistic quantified self (Fig. 1). In the context of RECALL we investigate such a holistic approach to strengthen and augment human memory.
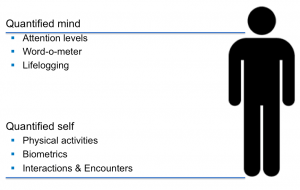
When talking about memory recording we distinguish between explicit and implicit recording: Whenever we stop to capture the moment by taking a picture or video, we explicitly take a snapshot of that moment. Hence, explicit recording entails the conscious attempt to record a memory. Posting on Twitter, Facebook or Instagram would be considered a conscious effort to communicate a thought, experience or feeling. When users make the conscious decision to record a moment or content item, they should have an appropriate tool at hand to do so. We have been experimenting with different approaches concerning user dialogues that are user-triggered and walk the user through a sequence of tasks to be completed in order to record a moment.
With explicit memory recording there is, however, a trade-off between enjoying a moment by being fully focused at it, and making the decision to record it by taking out a device and go through a designated sequence of steps. Between un-obtrusiveness and memorability there is a sweat spot, we believe, which we are aiming to find.
To make sure the focus of attention is where it is needed – the current moment, the article currently being read – explicit tools for memory capture may not be the best solution as pointed out. The time required to take out the phone or camera, aim it at the object of interest and make that picture to retain that moment often times disrupts the enjoyment of it. Other times we only realize that a moment or detail was worth capturing when it has passed. Therefore we are looking at implicit ways of logging data that can be automatically collected from the user or the environment. Aforementioned devices, such as Fitbit, allow a constant logging of movement data. We can further infer from smartphone and app usage who a person interacted with over the course of a day, what events went by recorded by the calendar, or which locations were visited during the day.
Additionally, services like Facebook, Foursquare or Twitter provide us with APIs to retrieve user activities. From this data we can construct a summary of distinct events a person experienced over the course of a day, week, month or year, thereby creating digital memories that can be indexed, searched or browsed later on. Context awareness is highly beneficial as it allows us to infer what state a person is in or which application is currently pre-dominant on the user’s phone [5]. We are currently developing a modular technology platform that pulls information from all kinds of services and bundles them into a digital memory system.
Combining implicit and explicit data capture to end up with a life logging solution that comprises both physical and mental states of people requires tackling a number of challenges:
Meaningful Data
Meaningful data heavily relies on the data quality and the legitimacy of conclusions we draw from them. This is especially true for implicitly collected data, where inferential conclusions may be ambiguous.
Technology Constraints
There are constraints on what we can track when it comes to physiological and psychological activities. Despite the progress made regarding tracking physical data, tracking mental activities is still suffering from numerous limitations: eye trackers are used to capture users’ attention focus and Electroencephalography (EEG) is used to record the electrical activity along the scalp. Unfortunately, these techniques only give us a partial picture. Additionally, we still have to accept the fact that certain values are simply not attainable in a non-invasive way with current technologies (i.e. blood sugar levels, kidney values).
User Acceptance
We may design software as well as hardware in order to support human memory. In the end it is still up to the user and her willingness to actually use these technologies in order to decide whether they are feasible, effective, usable and fashionable. To reach user acceptance, these technologies will need to be unobtrusive, non-invasive and informative when it comes to the presentation of the data that they are logging.
Call to Action
Simply collecting data and inferring activities may not be enough. The way this data is processed and presented is crucial. Data alone is useless until we derive some meaning from it and give the user actual information about her state, progress and possible goals. Intelligent algorithms come into play when it comes to making meaning of data and deriving concrete user recommendations on how to change behavior regarding health practices, fitness or environmentally sustainable choices.
Concluding, there is always room for discussion whether holistic logging is a good idea in general. The moment we leave it up to algorithms which moments we are actually going to remember, brings up a range of ethical questions. Which memories should be attenuated?; which ones should better tried to be forgotten?; and is there forgetting in a system that nearly never runs out of memory space? These are the issues we are trying to explore through both technological prototypes and active discussions.
[1] Gemmell, J., Bell, G., and Lueder, R. Mylifebits: a personal database for everything. Communications of the ACM 49, 1 (2006).
[2] Hodges, S., Williams, L., Berry, E., Izadi, S., Srinivasan, J., Butler, A., Smyth, G., Kapur, N., and Wood, K. Sensecam: A retrospective memory aid. In Proceedings of UbiComp. 2006.
[3] Khan, A. M., Lee, Y.-K., Lee, S., and Kim, T.-S. Human activity recognition via an accelerometer-enabled-smartphone using kernel discriminant analysis. In Proceedings of FutureTech (2010).
[4] Kunze, K., Kawaichi, H., Yoshimura, K., and Kise, K. The wordometer–estimating thenumber of words read using document image retrieval and mobile eye tracking. In Proceedings of ICDAR (2013).
[5] Sahami Shirazi, A., Henze, N., Dingler, T., Kunze, K., and Schmidt, A. Upright or sideways?: Analysis of smartphone postures in the wild. In Proceedings of MobileHCI (2013).